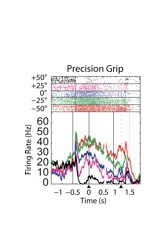
Cortical representation of grasping actions
Primate hand movements are complex and highly cognitive forms of behavior. Appropriate planning of hand movements requires the integration of sensory information with internal (volitional and memory) signals in order to generate appropriate hand actions. We investigate how hand movements are generated in the primate brain, specifically in the higher order brain areas of the parietal (anterior intraparietal area; AIP) and the premotor cortex (ventral premotor area, i.e., area F5). We perform dual-area recordings in behaving animals (spiking activity and local field potential (LFP)) in order to characterize the role of these areas for sensorimotor transformation and decision making related to hand grasping. This will shed new light on how hand movements are generated in the brain.
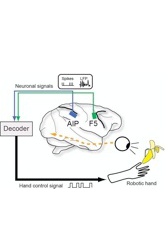
Real-time decoding of hand movements
Using our current understanding of how hand movements are represented in motor, premotor, and parietal brain areas, we are developing brain-machine interfaces that can read out such movement intentions to control robotic devices in real-time. For this we employ permanently implanted electrode arrays that can read out cortical signals simultaneously from about 100 channels or more. Using dedicated analysis software, these signals are then decoded in real-time to make predictions on up-coming grasping actions, which are then fed back to the subject and used to control robotic hands. Such systems could be useful for future applications aiming to restore hand function in paralyzed patients.
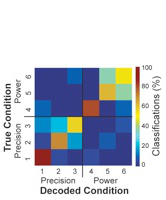
Optimal decoding from neural ensembles
The brain represents information in a distributed fashion. Neural interfaces that attempt to read out neural information from the brain therefore have to take the multi-variate nature of these signals into account. Using neural data from decoding experiments from multiple cortical areas, we investigate how prediction algorithms can be improved by taking timing information, the multi-variate nature of the signals, and various classifier methods into account. Such improved decoding methods will advance the efficiency of neural interfaces.
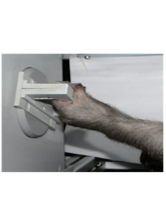
Grasp kinematics
An important aspect of the neural activity related to hand grasping is the representation of hand kinematics. To understand the neural representation of hand kinematics, neural activity has to be correlated with hand kinematic data. We are currently developing a hand tracking system that can monitor three-dimensional hand and finger movements in small primates during object grasping. Such a system is essential to understand the neural representation of hand and finger movements.
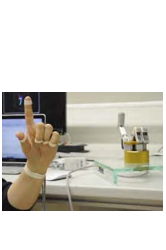
Hand robotics
Neural interfaces for grasping need robots to execute the decoded hand actions. While virtual environment solutions are possible at an initial stage, robotic hands provide far superior feedback and interaction with the real world. Robotic hand control integrates command signals from the neural interface and with sensory information from the robot to generate grasping actions. Furthermore, proprioceptive (sensory) information can be sent to the brain with an additional sensory interface to provide robotic feedback independent of vision. Such bi-directional neural interfaces could therefore lead to considerably improved neuro-prosthetic systems.

Investigation of the primate hand grasping network with pathway-specific neuro-optogenetics
Drawing not only correlative conclusions, but causal links, between the specific neural components of the hand grasping network involved in the planning and executing of hand grasping movements is essential for the development of neuroprosthetic devices. Accordingly, we are currently applying the emerging approach of optogenetics on the grasping network of behaving monkeys. Neuro-optogenetic tools have recently been established in primates and allow the precise manipulation of neuronal activity with unprecedented temporal, spatial, and cell-type specificity. We combine several behavioral and neural recording methods including neuro-optogenetics, intracortical electrophysiological recordings, and hand kinematic tracking in macaque monkeys while performing a delayed grasping task. We examine the effects of optogenetical stimulation on the local and remote, but directly connected, neuronal activity as well as on hand grasping behavior. Results of this study will likely provide significant new insights on the functional contributions of the fronto-parietal hand grasping network and their causal interconnections. Ultimately, results from these studies could contribute to the development of improved neuroprostheses for impaired patients and the translation of optogenetic methods into the realm of clinical trials for humans.
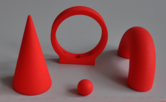
Influence of tactile information on hand motions
Besides investigating how grasping movements are generated in the brain, we are also interested in the influence of different senses on hand movements. For this, we present different structures and objects to non-human primates that they then touch. During this tactile investigation we record brain activity in relevant brain areas, like the somatosensory cortex (S1, i.e. involved in the sense of touch). This helps us to better understand how the brain perceives texture information.
In another experiment we investigate whether there is a difference on how the brain generates grasping movements when an object was only seen or only felt. The objects, for example a small sphere, are either illuminated or stay hidden in the dark and the non-human primates are only allowed to touch, but not see them before the objects are lifted up.

Decoding of hand movement for brain computer interfaces
How does the brain produce movement? How do billions of brain cells harmonize the activity of hundreds of muscles to produce the movement basic for our daily life such as grasping? This question has fascinated scientist and engineers for millenia, but beyond that it has become crucial for medical advance: in cases such as paralysis and extreme cases such as locked-in syndrome, a better understanding of how the brain produces motion would allow engineers to develop devices that can recover some mobility and restore a patient´s social connection. We aim to understand how to brain produces the necessary signals to control arm movements. Specially, we investigate the crucial question: how can multiple parallel signals, such as the ones required for arm movement, be decoded fom the cerebral cortex to control an artificial device? For this we perform experiments in primate while they perform real and virtual grasps via a brain computer interface to understand the neural population activity patterns that coordinate sequential actions required for grasping.
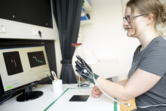
Investigating Human Hand Kinematic
Humans are using their hands effortlessly for daily tasks. The loss of a hand has a severe impact on one´s life and current prostheses are only giving relatively limited functions back. For contributing to a more natural like hand prothesis for the future, we investigate hand kinematics in healthy human subjects. Therefore we employed a data glove system that can track essentially all hand and finger joints (27 degrees of freedom) to investigate hand kinematics and laterality differences between both hands. Particular research topics include human handedness and a better understanding of the impact of the loss of the dominant or non-dominant hand. Furthermore, we aim to increase our knowledge about daily environment interactions which might be challenging for amputees.
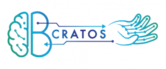
EU funds cooperation project to connect brain, body and machine
Development of a communication platform in the body to control prostheses
The collaborative project B-CRATOS ("Wireless Brain-Connect inteRfAce TO machineS") aims to control prostheses or "smart" devices by the power of thought. For this purpose, a battery- and wireless high-speed communication platform is to be integrated in the body to connect the nervous system with signal systems and thus stimulate various functions of, for example, prostheses. The project, coordinated by Sweden's Uppsala University, also involves besides five European partners including universities, companies and institutes, the the German Primate Center (DPZ) to test the new technology in non-human primates. The highly ambitious project, which combines expertise and cutting-edge technologies from the fields of novel wireless communication, neuroscience, bionics, artificial intelligence (AI) and sensor technology, will receive 4.5 million euros in EU funding over the next four years.